Improving mill operator and production efficiency via Artificial Intelligence (AI) and Machine learning.
Real-time finished quality measurements (i.e., STFI, Mullen, tensile, wet tear, etc.) can significantly improve paper mill operations and decision making. However, real-time physical sensors do not exist for these quality parameters. The only way to achieve real-time insights is through mathematical predictions generated using artificial intelligence and machine learning. Solenis’ Optix applied intelligence provides enhanced process visibility with an analytics platform that uncovers mill improvement opportunities not previously possible.
With increasing availability of instrumentation and use of centralized data historians, mills are collecting vast amounts of data that provide them with ever-increasing visibility into their processes. However, the current data analytic tools are mostly backward looking and have specific limitations in highly variable, continuous-process manufacturing like the paper industry. The typical mill can have as many as 10,000 data historian tags that can be associated through highly complex, multidimensional variable relationships. Today’s tools simply cannot handle the computations required to evaluate this level of complexity and nonlinearity in real time.
Predictive analytics supported by machine learning can provide real-time quality measures that remain robust and accurate in the face of changing machine conditions. These adaptive quality “soft sensors” allow for more informed, on-the-fly process changes, rapid change detection, and process control optimization without requiring periodic model tuning.
The solution
Optix applied intelligence is a novel adaptive analytics platform built with the latest artificial intelligence (AI) and machine learning capabilities available today. Optix was developed to provide an advanced digital service for the mill of the future and it can be tailored to every mill’s unique processes. Using process data, physical paper measurements of the critical quality parameters and papermaking process knowledge, Solenis applies robust data science techniques to turn complex, multidimensional relationships into an accurate, real-time, adaptive “soft sensor.” Unlike other data analytic tools, Optix does not require time-consuming data interpretation and quickly provides an actionable solution. The resulting real-time predictive and prescriptive analytics enable a step change improvement in mill optimization not previously feasible.
Solenis’ data scientists carefully evaluate mill process data using sophisticated data collection, cleaning and mining techniques. Advanced data mining practices, such as multiple regression and causal networks, reveal multidimensional relationships between the response and predictors that are not easily identified any other way (figure 1). Solenis employs a proprietary screening methodology that allows Optix to focus on the most important tags needed to drive the machine-learning, predictive platform.


The predictive model delivered by Optix provides a real-time, mathematically driven measurement for paper quality metrics. While the traditional method of laboratory testing key quality parameters only provides a quality measurement every reel turn-up or less frequently, Optix provides high-frequency data every 15 to 30 seconds. Optix real-time prediction provides significantly more insight into the primary quality parameter than physical lab measurements alone (figure 2). Papermakers can have a real-time, machine-direction quality profile for the entire length of the reel.

Machine learning and deep learning
It is crucial for analytics platforms to adapt to the ever-changing environment of the papermaking process. Solenis’ predictive models incorporating machine learning adapt to changes in the process by learning from observations and interactions. This information drives shifts in the predictive model aimed at maintaining prediction accuracy. This level of machine learning is built into the platform, which is why Optix measurements remain robust and accurate in the face of changing machine conditions. A static predictive model can lose prediction accuracy over time, whereas the machine learning algorithms constantly tune predictive models and preserve prediction accuracy in real time (figure 3). Each Optix model is also reinforced with a self-diagnostic prediction accuracy monitor, which calculates the difference between the prediction and actual value of the quality measurement.
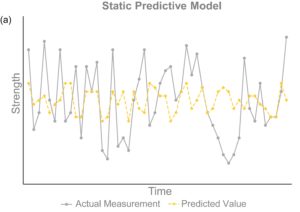
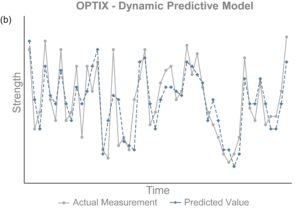
A successful predictive model can be further transformed into a prescriptive analytics tool. Using deep learning and advanced data analytic methods, Solenis developed a prescriptive recommendation engine. Optix prescribes real-time actionable insights for optimizing the papermaking process. Operators can use data-driven recommendations to maintain manufacturing conditions, target optimized machine efficiency, or focus on cost-saving objectives.
The development and application of adaptable predictive and prescriptive analytics requires a team of data scientists and subject matter experts. The Solenis service model includes access to a full team of experts, reducing the necessity to dedicate additional mill resources. By providing a monthly subscription service, financial risks are minimized, thus circumventing capital expenditures. Solenis’ rapid implementation process ensures that each mill’s predictive model can be developed and delivered in as little as 12 weeks.
Return on investment
Optix enables a significant error reduction of the finished quality parameter (figure 4). When the prediction is used in place of the lab measurements to make quality control decisions, the system variability decreases because the testing error is no longer transferred into the process.
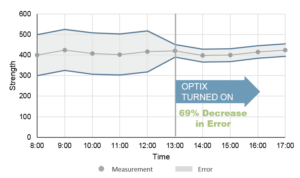
In real time, operators can see the impact of process adjustments to the finished quality parameters allowing for optimization of key process variables. A customer was able to reduce basis weight by approximately 1.5% while driving primary strength quality parameter to an optimized state (figure 5). The basis weight adjustment resulted in an estimated annual fiber savings of approximately $450K.
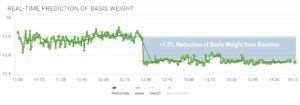
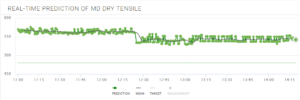
Real-time predictions become invaluable during critical process changes such as grade changes. By closely monitoring the impact of process adjustments on the finished quality parameter, rapid grade change is possible. The steady change possible when the operator utilizes papermaking process knowledge and the process visibility provided by Optix (figure 6). Additionally, operators can utilize Optix real-time predictions to proactively make process changes to avoid off-grade paper. Ultimately Optix encourages improved quality consistency resulting in less downgraded or re-pulped products.
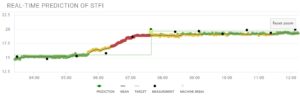
Conclusion
Optix Applied Intelligence is a comprehensive predictive and prescriptive analytics tool for the paper industry. By utilizing machine-learning, the platform remains accurate and robust in continuous process manufacturing. The real-time, actionable quality measures generated by the soft sensors allow operators to optimize paper production while reduce costs.